Data on inequalities: approaches, issues and challenges
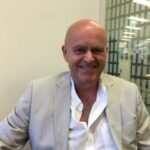
Background
This keynote speech was given by Richard Laux at the Government Statistical Service (GSS) Conference 2023 on Wednesday 8 November 2023.
Richard is the Head of the Equality Data and Analysis Division in the Equality Hub. He is also the Chief Statistician in the Cabinet Office.
Introduction
The title of today’s conference is “using statistics to build a more inclusive world”. My job is entirely about statistics and their use in helping to build an inclusive world.
It’s about the statistics we need to tell us about people with different characteristics, like their ethnic group and whether they have a disability.
My team’s work supports the Minister for Women and Equalities.
I know from discussions I have, that people inside and outside of government put a great deal of value on data and evidence about inequalities. And they want it to be known that they value this information. There is a big emphasis on this area being “data-driven”.
This emphasis is different from other areas I have worked on. No-one expects the Bank of England to explain that in setting interest rates they look at the data. It’s a given. But people do say this about addressing inequalities.
Maybe it’s because ‘inequalities’ is hotly contested and political, so everyone wants to give the impression that their political response is uniquely correct because it’s ‘based on the evidence’?
Or maybe it reflects a general recognition that the cost of inequalities can be so great that addressing inequalities genuinely requires an evidence-based approach.
I will raise some of these issues today. I will also talk about:
- concepts and definitions — for example, what do we mean by equality data? Does equality data even exist?
- analytical approaches and some aspects of data that we analysts need to consider
- different approaches to the analysis of inequalities
I will finish with a few words about addressing future challenges.
Concepts and definitions
I will start with some concepts, definitions, and terminology.
This conference is about inclusivity and inclusive statistics. By inclusive statistics we mean that we collect and publish data for all people, regardless of their location, ethnicity, gender, age, or other characteristics.
It’s also about diversity. This means involving people from a range of different backgrounds and characteristics.
It’s likely that the conference will also consider disparities. These are differences in level or treatment, especially ones seen as unfair.
The conference might also touch on inequity. This is a lack of fairness or justice.
Finally, the conference might consider discrimination. This is one of several potential reasons for disparities. It’s an ‘input’ rather than an ‘outcome’. It can be difficult to show using the results of traditional surveys.
I have two thoughts around this.
Firstly, these are related or similar concepts. From a policy and political context, it’s really important to be clear on what’s being talked about. This applies in our analytical world too. We need to be clear about our concepts so that we can measure and describe data confidently.
Secondly, ‘disparity’ and ‘inequality’ are words that both describe differences. But a disparity is ‘just’ an unfair difference between two variables, while an inequality is unfair, avoidable, and systematic. An equality is somehow ‘bad’ and by implication ought to be changed.
So, when we describe disparities as “inequalities”, we’re at least implicitly taking a political or moral position. The term is not neutral. That’s not a problem in itself. We’re civil servants addressing issues that our Ministers want to see dealt with. But it’s worth remembering that we’re working in a political environment and on topics where there’s a high degree of subjectivity.
I’m going to use the words ‘equality’ and ‘inequality’ in my speech. This is because most of my work is about understanding the differences in size, degree, or circumstances between people, with the aim of doing something to reduce the differences.
What we mean by equality data
I talk about ‘equality data’ but I’m not sure there is such a thing. Instead, we have data about a range of social, economic, and environmental conditions.
“Equality” comes in when we start analysing the data and trying to answer the questions that the data suggest to us.
This is when we need to be clear about who and what we are measuring and comparing. For my team, most data or comparisons are about differences between groups of people.
But there are also spatial inequalities. Sometimes spatial equalities are phenomena like rainfall or pollution levels. But when we talk about spatial inequalities we often mean socio-economic inequalities analysed by geography.
These are much-loved by the media. The combination of locality and injustice appeals to deep-rooted instincts. For example, the media often report on things like ‘postcode lotteries’ in access to health services and choice of schools.
Sometimes we overlay information about where people live or work by other individual characteristics like age or ethnicity.
“Levelling Up” is about place-based inequalities. It’s about tackling inequalities by concentrating on the characteristics of different areas, rather than on the characteristics of the people in those areas. This is a nice illustration of the importance of looking at different types of inequalities and of looking at inequalities in different ways.
I started this section by questioning “equalities data” as a concept. Hopefully I have clarified my position. For me it’s a shorthand for social, economic and environmental data analysed in ways that bring out inequalities between groups of people or areas.
Analytical approaches
Next I will talk about some of the analytical approaches we use to explore inequalities.
First, we have different types of comparative or descriptive analysis. These types of analysis might be relatively simple. For example, we might compare average earnings for men and women. It might also be easy to interpret this sort of analysis. But the analysis might suggest a misleading story. There might be underlying factors that we should consider. For example, we may need to think about the number of men and women in part-time and full-time jobs, and rates of pay for part-time and full-time workers.
This leads us to more complicated analysis. My team has been looking at changes in the relative disparity between stop and search rates for different ethnic groups in London and outside London since 2011.
Here we’re comparing figures in three different ways. We’re comparing the ethnic minority groups in relation to the white group, for different geographies, and over time. It’s complicated, but it can help us to understand why the national stop and search figures are as they are.
We sometimes call this intersectional analysis. This is an analysis of groups of people defined by multiple personal characteristics. It adds value to simpler sectoral analysis, which looks at outcomes for particular groups in isolation.
These are univariate and multivariate approaches to segmenting data. There are also increasingly complex statistical techniques, such as regression analysis, that can account for other factors and so help us to go beyond description into explanation. My team worked with the Office for National Statistics (ONS) last year to produce equality analysis across different areas of life in the UK using a regression analysis. That allowed us to compare the outcomes of different population groups across several topics including crime, mortality and life satisfaction. We introduced explanatory factors in sequence so we could see which ones affect the relationship between the population group and the outcome.
Data issues
I will talk about data issues now. None of these types of analyses exist in a political or social vacuum. There are always issues and context.
Context
One thing we need to understand is the historical context of inequalities. Regression analysis will generally tell us that socio-economic status plays a more significant role than ethnicity, in terms of disparities in outcomes. But often little attention is paid to why people from some ethnic groups are more likely to be in lower socio-economic groups than others. It’s unlikely that any policy intervention will be completely effective if we do not understand the historical context.
And there’s an extension of this point about historical context. The language we use about ethnicity and sex and gender is not fixed. It changes over time and will carry on evolving.
Language is also different in different places. For example, terminology about race in the USA is different from the terminology used here in the UK. Phrases like “People of Colour” and “indigenous” are generally not used in the UK, but are commonly used in the USA. Similarly, the word “Asian” in the USA refers to people from a different background than it tends to mean in the UK.
This means the ways we talk about personal characteristics are often dependent on time and place.
Some personal characteristics are also currently very politically sensitive. These include sex and gender identity.
Data quality
Another big issue is data quality. This is an important area of work for my team. There are some common challenges when we look at data about ethnicity, sexual orientation, disability, or other characteristics.
These include how to deal with small sample sizes. This is a challenge when we want to look at the Gypsy and Irish Traveller groups, for example, or people who are transgender. And it’s a very difficult challenge when we want to look at intersectional analysis or subgroups, which I mentioned before.
There’s a practical limit to analysis when you’re looking at these very small subgroups. Sample sizes, or numbers of people in some subgroups, can get very small, very quickly.
Another data quality issue is dealing with high levels of missing data about people’s characteristics. There’s a public good in collecting this sort of data because of the analysis it lets us do. But, apart from the Census, most data collections are voluntary and some suffer from a lot of non-response to demographic questions.
We also need to follow the General Data Protection Regulation (GDPR). We must have a need for the data we collect.
There can be tension here between:
- our role as analysts who want as much data as possible to do as much good as possible
- the need to allow people not to respond to our questions
This is an unresolved issue for me, maybe it needs ONS’s corporate heft to get to the bottom of it. Or maybe there’s just not a way to resolve this issue.
We also want data providers to use harmonised categories – standardised ways of presenting and collecting data across Government – as much as possible. This can be difficult if users need data about groups which are not part of a harmonised standard. In recent years there has been a lot of pressure to include ‘Sikh’ as an ethnic group, for example.
There can be sensitivity around harmonised standards and categorisation. Some topics, like people’s occupations or transport patterns, are important but generally regarded as neutral or uncontentious. Other topics, like ethnicity, are much better understood and discussed more often than 20 years ago, but there are still sensitivities around terminology and labelling.
Some people do not like to be categorised. We often present ethnicity data for Asian, Black, Mixed, White, and Other. But most people do not think their ethnic origin is best described as “Other”. This is about as non-inclusive as it gets. Other people do want to be categorised but in ways that we do not support, like the Sikh group being an ethnic category, mentioned before.
We face the classic statistical quality challenge of trade-off when we consider the benefits and challenges of making changes to what we ask respondents about. In some areas of statistics we’re used to balancing the speed of publication with the accuracy of our results. In this case the trade-off is between data continuity and the relevance of data.
We could change the ethnicity categories every year to be really relevant and inclusive. But if we did that we would not have a consistent time series to see whether disparities between ethnic groups were reducing. But if we do not change the ethnicity categories often enough, they might not reflect how people think about themselves and their ethnicity. There is a very fine balance in these decisions.
Data gaps
My next set of data issues is about gaps in the evidence base. What do we not have data about?
There are lots of examples. For example, we do not have comprehensive data about disability and impairment, or regular data about people’s religions.
My team has recently looked at how we might get better data about online harm and who experiences it. This includes more data about online misinformation and more regular, comparable data about online hate crimes.
Data on parents’ occupations would be incredibly valuable. It is crucial in supporting social mobility analysis.
To understand what data we need in the equality space we need to understand our users’ needs, and understand new areas of social and policy interest. Sometimes this policy interest might be in improving outcomes for specific groups in the population. Sometimes it might be thinking about policies that can improve outcomes for everyone: “a rising tide raises all boats”.
Whichever approach is being taken it needs data that is fit for purpose and disaggregated as much as possible. For example, data could be separated to give detailed information about ethnic groups, sexual orientations, or types of impairment.
Data linkage
In this section about data issues I have talked about the importance of historical context, and traditional statistical concerns about data quality and data gaps. Now I want to mention a very current issue. It helps with both improving data quality and filling gaps in the evidence base. This issue is data linkage.
The ability to link data sets continues to be hugely important. For example, during the COVID-19 pandemic, the ONS developed the Public Health Data Asset, which linked:
- the 2011 Census
- death registrations data
- GP data
It was the basis of several ONS research projects, including the publication of information about differences between deaths involving COVID-19 for different ethnic groups.
This was really important for my team working in this area at the time. This was because ethnicity is not collected during death registration. The ONS work in this area helped us to understand how COVID-19 was affecting different ethnic groups.
I do think that it can be difficult for others to get data linked in practice. In general, our data sharing and linkage culture feels like it’s on the basis of exception. It does not yet seem to be enthusiastic. We do not “default to linkage” as a principle. We are not bold.
The tension I mentioned before between collecting as much data as possible and GDPR is here too. By ‘default’, I do not mean that we should necessarily link every dataset to every other dataset. We still need to be mindful of the legal issues around data linkage. Again, this means following the GDPR and the Public Sector Equality Duty. But more data linkage could create new opportunities for analysis. These might uncover further, unknown disparities or issues that we might address. A more permissive approach to data linkage, combined with greater use of data mining approaches, might uncover truths and patterns that we cannot currently see.
Related to this, ONS has recently finished consulting on the future of population and migration statistics. This is really important as it will inform the decision about whether to run a 2031 census, or whether administrative and other data can provide what stakeholders need.
In my world that means data about people’s characteristics. The approach being proposed would seem to provide data that is not in the census, if it is possible to ensure that the data quality is sufficient. For example, this could include data about income by ethnicity provided on a regular basis.
The point about data quality is an important caveat. ONS has shown there are lots of inconsistencies between people’s data on health administrative datasets and their census response. Much of this is understandable. The datasets do not cover the whole population, and not everyone can be linked between the census and the administrative datasets. And when they are linked, the person might have given different responses in the census and administrative dataset. People might also give a different ethnic group in different administrative datasets. So, there is a lot to overcome here for ONS and others before this particular vision of the future can be made real.
The data issues I have been talking about apply to all the countries of the UK. Each of the devolved administrations, and the UK Government, have their own policy challenges in relation to different equality groups. But many of the challenges relating to data collection, analysis, and reporting are largely the same.
Different approaches to analysis and thinking about data
The issues I have been talking about might mean we want to think differently about ‘inequalities data’.
I think we need more of an emphasis on the economic analysis of inequalities. This might be something ONS could provide by examining its data holdings from a micro-economic perspective. And at the macro-economic scale, by looking at the economic benefits and making the economic case for equality.
And perhaps we need more emphasis on the qualitative to complement what we do quantitatively.
Co-design and qualitative research are essential. We should be including people from the populations we are analysing when we’re selecting our research questions, as well as in the design of surveys. This can help in providing statistics that matter to that population. It can make the statistics more relevant and inclusive.
Secondly, quantitative data cannot tell us everything we need to know about inequalities between different groups of people. They can help us understand the scale of the problem. They can also point to the factors associated with the problems. But we cannot ever get a true sense of what is actually happening in society by using statistics alone.
And in parallel we need better ways of explaining the merits of qualitative data to users.
This means I would urge more qualitative thinking around equalities. But also more mixed-methods work, so I would encourage combining quantitative and qualitative approaches. I mentioned earlier that it’s very difficult to demonstrate discrimination using traditional population surveys. Mixed methods approaches can help with that.
So I’m proposing more analysis of different types. But who’s going to do it? Those of us in government and the wider public sector have limited time to be doing much of this analysis. I think there’s scope to work more closely with research and academic institutes. There are great swathes of research and data collection available externally. When we have worked with outside researchers and academics in the Equality Hub, the results and sense of win-win have been great.
Addressing future challenges
Throughout my speech today, I have described some of the challenges associated with equalities data.
I have talked about:
- understanding the historical context and data quality issues like linkage, filling gaps and harmonisation
- putting more emphasis on economics
- using qualitative and quantitative information together
I have also proposed that we work with academics and researchers more regularly.
I think addressing these challenges is about the right leadership.
Strong leadership in partnership with other colleagues – and of course, dedicated resources – can:
- help develop partnerships with the analytical community outside government
- bring departments together to provide a coherent economic emphasis on equalities as well as coordinating social analysis more effectively
- enable us to concentrate on methodological and data quality improvements, better ways of linking data, and user engagement to identify priorities
The Cabinet Office, including my team in the Equality Hub, have been working closely with ONS to provide leadership and will continue to do so. We will continue to build on the emphasis on equality data from Professor Sir Ian Diamond. This has been most visible in the form of the Inclusive Data Taskforce implementation plan. It’s helped us progress, with departments and other organisations working towards their actions in the plan.
But now I would argue we need to ramp up our efforts. We need to build on what we have achieved in the last few years, organisationally and technically, so that we’re able to develop our work to make greater use of administrative data. By this we will ensure policy-makers can continue to take a data-driven approach in their work on equalities.
The final thought I would like to leave with you is related to leadership. What more might ONS and the Cabinet Office do to help secure the future of data and analysis about equalities?
And what more might you do in other Departments either formally, for example in the spirit of the Inclusive Data Taskforce Actions, or informally, for example in the spirit of ‘communities of interest’, to progress this agenda?