Status of the document
This paper summarises key insights from the Office for National Statistics (ONS) programme of work that was funded by the Wellcome Trust to investigate the consistency and quality of ethnicity data within NHS health administrative data sources.
We are grateful for the contribution of the Wellcome Trust, Expert Review Panel and ONS colleagues who guided the analyses and direction of the programme of work. The Expert Review Panel comprised colleagues from NHS England, Office for Health Improvement and Disparities, University of Leicester, Leicester Real World Evidence Unit, Centre for Ethnic Health Research, Swansea University, De Montfort University, The King’s Fund, The Health Foundation, and Genomics England.
Scope
This paper collates the collective findings from Office for National Statistics (ONS) analyses examining the consistency and quality of ethnicity coding in health administrative data sources, which was funded by the Wellcome Trust.
In this paper, we acknowledge the previous research and progress achieved by other organisations investigating this area. We also acknowledge partner agencies who are working toward improving the understanding, collection methods, terminology, standardisation, and quality for ethnicity data collected in health administrative data sources. We summarise the findings of our analyses, which was interpreted by experts from different disciplines, and propose a set of learning points for deriving ethnic group data when using primary and secondary health administrative data sources. The learning points in this paper only apply to General Practice Extraction Service (primary care) and Hospital Episodes Statistics (secondary care) data. Whilst we acknowledge that some learning points we provide and wider findings from our analyses may be applicable to other administrative datasets, we do not directly provide advice for them as they were not included in our original analyses. This paper compliments wider research towards developing population statistics by ethnic group using administrative data as part of the future population and migration statistics system as detailed in our dashboard on the topic. The UK Statistics Authority (UKSA) will be publishing a recommendation to government regarding our proposals later this year.
This document is:
- a set of learning points for people to consider when working with and analysing ethnicity data
- a collation of findings from an ONS project assessing the quality of ethnicity data, taking into consideration previous learning points from organisations, and interpreted in the context of analysts and organisations who utilise ethnicity data for analysis
- a starting point to be built upon, taken forward, and improved by others
- a framework to complement and build upon department specific learning and procedures
This document is not:
- a definitive or authoritative guide for analysts and organisations to follow blindly without consideration of their specific analytical and research context
- an exhaustive list of all scenarios someone working with ethnicity data may encounter
Executive summary of main learning points
The main learning points in this paper for people working with ethnicity data in GPES and HES include:
- Learning point 1 – understanding the differences in ethnicity data in GPES and HES
- Learning point 2 – aggregation of ethnic categories
- Learning point 3 – methods for allocating an individual’s ethnic category from their entire electronic health record history
- Learning point 4 – whether reallocating ethnic categories is suitable and methods for reallocating ethnic categories
- Learning point 5 – how to prioritise ethnicity data from different sub-data sources and apply a hierarchy for data sources
- Learning point 6 – pitfalls of missing ethnicity data
Background
Health administrative data sources
For analysts working with health administrative data sources, it is important to recognise the significance of ethnicity data in informing health research, policy development, and resource allocation. Ethnicity data within these datasets play a crucial role in understanding health disparities, identifying vulnerable populations, and tailoring healthcare services to meet diverse needs effectively.
Using ethnicity data from health administrative data sources can however, present unique challenges and considerations that analysts must address to ensure the accuracy and completeness, and subsequent reliability, of such data. In this paper, we aim to provide analysts with practical insights and learning for handling ethnicity data from these sources, focused on the most utilised primary and secondary care data in the UK. These proposed learning points, along with previous research, can allow analysts to improve the quality and utility of their analyses, while minimising the potential for biases and errors inherent in ethnicity coding within health administrative data.
The two health administrative data sources which this paper is based upon are:
- General Practice Extraction Service (GPES) – the learning points which this paper proposes are based on analyses which utilised the General Practice Extraction Service (GPES) Data for Pandemic Planning and Research (GDPPR), which is an extract of GPES data and will be referred to as GPES hereafter (the learning points in this paper will be applicable to GPES data)
- Hospital Episode Statistics (HES) – full HES extract used
These health administrative data sources were chosen because they are the two most used national health datasets and include near population-wide coverage of England. They, or publicly available extracts of these data sources, are often used to provide national-level insights into several aspects of England’s health service and for health research within academia, Government, NHS England (NHSE) and health organisations. It is acknowledged that there are other health administrative data sources available to researchers, but for the reasons stated above, this paper only provides learning points for ethnicity data within GPES and HES. It is likely that some of our findings and learnings may be applicable to other administrative data sources, however.
Not all analysts or health and research organisations have access to the same data sources. Ethnicity data collected in GPES and HES can sometimes be recorded differently. This therefore means that analysts may potentially produce slightly different estimates regarding ethnicity when using GPES and HES, and potentially when using other health data sources too. The differences and representativeness in ethnicity data between data sources may be related to inherent differences between the health data sources. These may include inconsistencies in the ethnic categories available, data collection methods/standards, the circumstances in which the data are collected or recorded, and differing age profiles and population coverage between the data sources.
For example, HES data has an older median age vs GPES data given that younger demographics are less likely to be admitted to hospital and engage with secondary care providers. GPES data is more likely to have higher population coverage, given more people will engage with primary care services than secondary care services. The environment in which data is collected may also have an effect on the quality of the data. In a hospital or emergency care environment, recording an individual’s ethnicity may not be a priority, whereas within general practice there may be more opportunity to collect this data.
Based on the findings of the Wellcome Trust funded programme of work, we propose a set of learning points to include in a framework and guide the use of ethnicity data in GPES and HES for analysts.
Main learning points
Learning point 1: An overview of the differences in ethnicity data in GPES and HES data sources
It is important to understand the differences in ethnicity data between health administrative data sources. The terminology regarding ethnicity data differs between GPES and HES (and Census 2021, which we used in our analyses). We used Census 2021 data as a comparator in our analysis because it is widely regarded as the most reliable ethnicity data available, given it is self-reported and engagement is mandatory. Census 2021 includes 19 ethnic categories, including a newly implemented Roma category. GPES has 18 ethnic categories (GPES categories are based on the 2011 Census). By contrast, HES data only contains 16 ethnic categories and does not include “White: Gypsy or Irish Traveller” or “Other ethnic group: Arab” categories. The HES categories were updated in April 2001 to represent the ethnic categories as defined in the 2001 Census. In the 2011 Census, the Chinese ethnic category moved from the “Other” ethnic category to the “Asian” ethnic category, and new groups for “Gypsy or Irish Traveller” and “Arab” were added. In GPES and HES health administrative data sources, the Chinese ethnic category is still within the “Other” ethnic group. The lists below show the differences in terminology for ethnic category between the data sources.
Ethnic categories available in Census 2021, GPES and HES data sources
The format of the two data sources differs. HES contains three individual subsets of data, all of which contain information on ethnicity. These are:
- Admitted Patient Care (APC)
- Accident and Emergency (A&E) and Emergency Care Dataset (ECDS)
- Outpatients (OP)
Within these sub-datasets, ethnicity data is stored as the 16 ethnic categories detailed below.
GPES is made up of patient and journal tables, which both contain ethnicity information. The ethnicity information within journal tables is recorded using Systematized Nomenclature of Medicine Clinical Terms (SNOMED CT) health terminology. Ethnicity SNOMED codes are more detailed than the 18 ethnic categories detailed above. On the NHS digital git repository, there are 489 SNOMED codes for ethnicity in total. These are mapped to the 18 ethnic categories using an NHS England mapping. The ethnicity information within patient tables is recorded using 18 ethnic categories.
Distributions of ethnic categories within Census 2021, GPES and HES data sources (using both the recency (most recent) and modal (most common) methodologies to derive ethnicity for GPES and HES) are detailed below.
Census 2021
- White: English/Welsh/Scottish/Northern Irish/British
- White: Irish
- White: Gypsy or Irish Traveller
- White: Roma
- White: Other White
- Mixed/multiple ethnic groups: White and Black Caribbean
- Mixed/multiple ethnic groups: White and Black African
- Mixed/multiple ethnic groups: White and Asian
- Mixed/multiple ethnic groups: Other Mixed
- Asian/Asian British: Indian
- Asian/Asian British: Pakistani
- Asian/Asian British: Bangladeshi
- Asian/Asian British: Chinese
- Asian/Asian British: Other Asian
- Black/African/Caribbean/Black British: African
- Black/African/Caribbean/Black British: Caribbean
- Black/African/Caribbean/Black British: Other Black
- Other ethnic group: Arab
- Other ethnic group: Any other ethnic group
GPES
- British
- Irish
- Traveller
- Any other White background
- White and Black Caribbean
- White and Black African
- White and Asian
- Any other Mixed background
- Indian
- Pakistani
- Bangladeshi
- Chinese
- Any other Asian background
- African
- Caribbean
- Any other Black background
- Arab
- Any other ethnic group
HES
- British (White)
- Irish (White)
- Any other White background
- White and Black Caribbean (Mixed)
- White and Black African (Mixed)
- White and Asian (Mixed)
- Any other Mixed background
- Indian (Asian or Asian British)
- Pakistani (Asian or Asian British)
- Bangladeshi (Asian or Asian British)
- Chinese (Other ethnic group)
- Any other Asian background
- African (Black or Black British)
- Caribbean (Black or Black British)
- Any other Black background
- Any other ethnic group
Table 1: Distributions of ethnic categories within Census 2021, GPES and HES data sources (using both the most recent and most common methodologies to derive ethnicity for GPES and HES) | |||||
Ethnic Category | Census 2021 Millions n (%) | GPES-modal Millions n (%) | GPES-recency Millions n (%) | HES-modal Millions n (%) | HES-recency Millions n (%) |
---|---|---|---|---|---|
White British | 38.5 (75) | 30.2 (69.5) | 31 (71.1) | 28.5 (59.7) | 28.5 (59.7) |
Other White | 3.1 (6) | 3.3 (7.6) | 3.9 (9) | 2.2 (4.7) | 2.3 (4.9) |
Indian | 1.6 (3.2) | 1.3 (3.1) | 1.4 (3.2) | 1 (2) | 0.9 (2) |
Pakistani | 1.4 (2.7) | 1.1 (2.6) | 1.2 (2.7) | 0.9 (1.9) | 0.9 (1.9) |
Black African | 1.2 (2.3) | 0.9 (2) | 0.8 (1.9) | 0.6 (1.4) | 0.7 (1.4) |
Other Asian | 0.8 (1.6) | 0.7 (1.6) | 0.8 (1.8) | 0.6 (1.3) | 0.7 (1.4) |
Any Other Ethnic Group | 0.8 (1.5) | 0.5 (1.1) | 0.7 (1.5) | 0.9 (1.9) | 1.1 (2.4) |
Bangladeshi | 0.5 (1.1) | 0.4 (1) | 0.4 (1) | 0.3 (0.7) | 0.3 (0.7) |
Black Caribbean | 0.5 (1) | 0.3 (0.7) | 0.3 (0.7) | 0.3 (0.6) | 0.3 (0.6) |
Irish | 0.4 (0.9) | 0.2 (0.5) | 0.3 (0.6) | 0.2 (0.4) | 0.2 (0.4) |
White and Black Caribbean | 0.4 (0.8) | 0.2 (0.4) | 0.2 (0.5) | 0.2 (0.3) | 0.2 (0.3) |
White and Asian | 0.4 (0.8) | 0.1 (0.3) | 0.2 (0.4) | 0.1 (0.3) | 0.1 (0.3) |
Other Mixed | 0.4 (0.8) | 0.2 (0.5) | 0.3 (0.7) | 0.3 (0.6) | 0.3 (0.7) |
Chinese | 0.3 (0.7) | 0.2 (0.6) | 0.3 (0.6) | 0.2 (0.3) | 0.2 (0.3) |
Arab | 0.3 (0.5) | 0 (0.1) | 0 (0.1) | No data | No data |
Other Black | 0.2 (0.5) | 0.2 (0.4) | 0.3 (0.7) | 0.2 (0.5) | 0.3 (0.6) |
White and Black African | 0.2 (0.4) | 0.1 (0.2) | 0.2 (0.4) | 0.1 (0.2) | 0.1 (0.2) |
Roma | 0.1 (0.2) | No data | No data | No data | No data |
Gypsy or Irish Traveller | 0.1 (0.1) | 0 (0) | 0 (0.1) | No data | No data |
Not Stated | No data | 1 (2.2) | 1.1 (2.6) | 4.3 (9) | 5.7 (12) |
Unresolved | No data | 2.4 (5.6) | 0.1 (0.3) | 2 (4.3) | 0.2 (0.4) |
Not Known | No data | 0 (0) | 0 (0) | 4.8 (10) | 4.7 (9.9) |
Source: Quality of ethnicity data in health-related administrative data sources from the Office for National Statistics
For more details on the definitions of modal and recency, please see our below sections, published code, or previous analytical publications.
Table 1 shows the distribution of ethnicity recording in GPES and HES data sources in populations which are linked to Census 2021 for comparison, which is widely regarded to be the most reliable ethnicity data available given it is self-reported and engagement is mandatory. The distribution of the ethnic categories within GPES and HES differs. This is partly because of the different ethnic categories available within the data sources as described above. In addition, unlike censuses, which are designed with user experience in mind to ensure that individuals have the requisite information to make an appropriate selection, the recording of ethnicity in healthcare environments varies. Primary care recording varies, with most collecting self-report at registration, but an individual can ignore the question without penalty. Further, in both primary and secondary care environments, ethnicity can be recorded by clinicians or administrative staff with some assumptions, with data often carried forward from previous records that were also assumed. Further, residual categories, such as Not Stated and Not Known, can be assigned to an individual in GPES and HES. Therefore, given the different environments in which GPES and HES data collect ethnicity data, there are differences in the number of Not Stated and Not Known assignments.
Learning Point 1
There are differences between GPES and HES (and, between all health administrative data sources) and it is important to be aware of this before commencing any analysis or working with the ethnicity data. There are differences in the manner ethnicity data is collected, the ethnic categories available, the terminology used, the structure of the data, and the distribution of ethnic groups between the data sources. Without consideration of these points before working with ethnicity data, biases may be compounded.
Learning point 2: What level of ethnic category aggregation?
Analysts should use the most disaggregated ethnic groupings where possible. This is because using five-category ethnic groupings (or similar aggregated categories) can mask differences within ethnic groups. For example, rates of stroke being highest in Bangladeshi individuals, with rates in Indian and Pakistani individuals similar to all other ethnic groups. If these categories were grouped as South Asian, the rates of stroke would be diluted in the Bangladeshi group. It is acknowledged however, that this may not always be possible given small sample size in some ethnic groups, or when restricting the sample based on inclusion or exclusion criteria in data analysis. Disaggregating ethnic categories with small sample size, or with a low number of events within the groups, may cause statistical uncertainty when applying statistical modelling or disclosure control issues. Therefore, a pragmatic approach is required. It is at the discretion of the analyst and team to decide on the appropriate level of disaggregation.
We propose the following example of aggregations that could be applied. The disaggregation examples are based on analysis assessing the population size of certain ethnic groups and comparison in demographic, social, and health characteristics between certain ethnic groups using Census 2021 data. The 19 category ethnic grouping should be used where possible, with further aggregation options shown.
Figure 1. Diagram of ethnic group aggregation options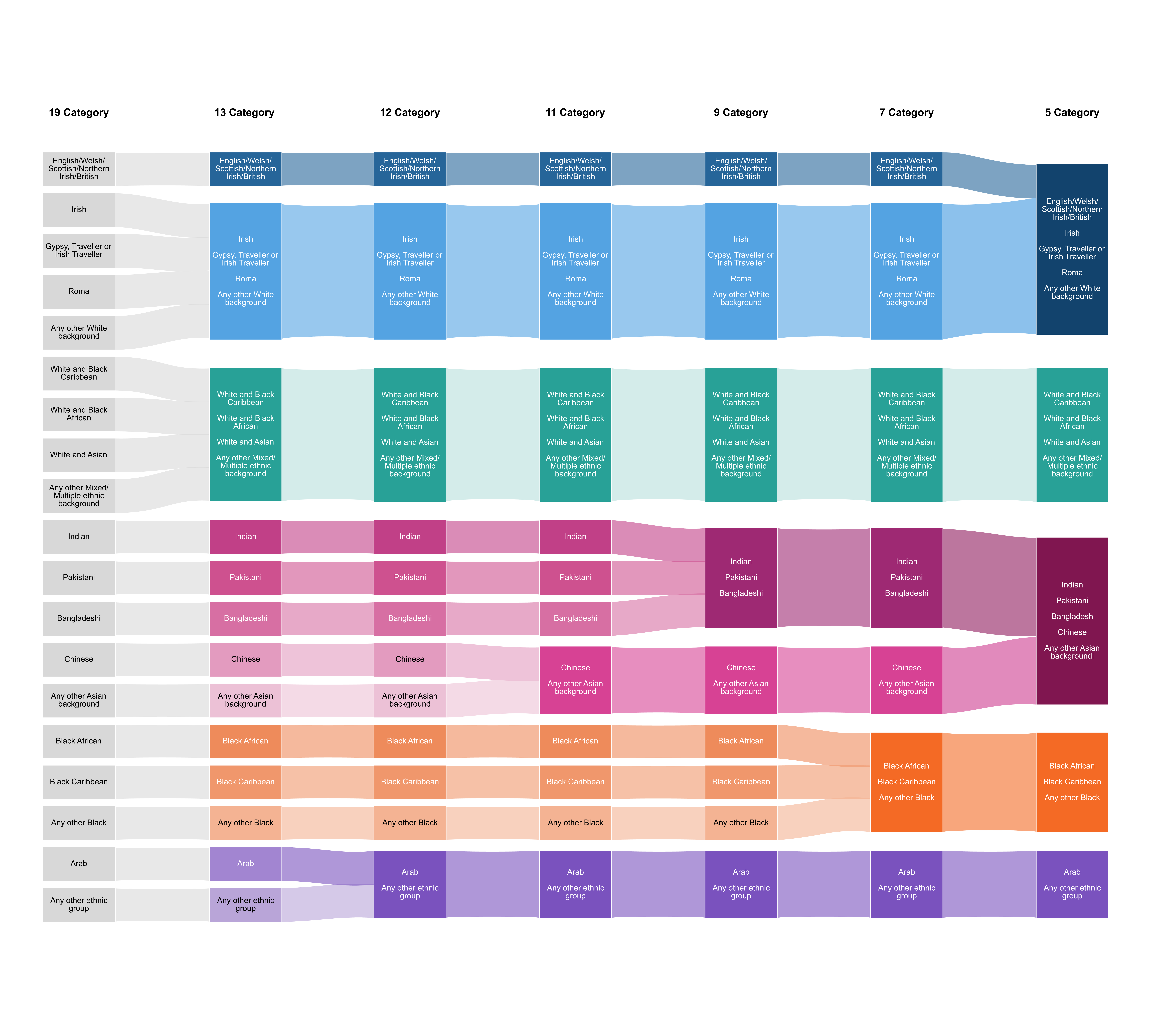
Learning Point 2
- Use 19 category ethnic groupings where possible. Where that is not possible due to statistical reliability, disclosure, or other relevant issues, we suggest using the highest level of disaggregation that is practical to limit statistical uncertainty or the influence of masking of ethnic differences in aggregated ethnic groups. Sometimes however, it may be required to use the least disaggregated (meaning the five-category) ethnic groupings, depending on the data available. Aggregating all ethnic minority groups together into a catch-all group (e.g. White vs all ethnic minorities combined) is not recommended because of the heterogeneity of the groups.
Learning point 3: Taking an individual’s most recent or most common ethnic category within their records
GPES and HES are both episodic datasets, meaning they contain information about all interactions a patient has with the relevant health service, so generally contain multiple records per patient. Within these data sources, some individuals have multiple recorded ethnicities. Therefore, there are different methods to allocate an individual an ethnic category.
Different major national health regulatory agencies derive ethnicity data differently. For example, NHS England (NHSE) currently uses the most recent ethnicity an individual has recorded to determine their ethnicity, while the Office for Health Improvement and Disparities (OHID) use the most common ethnicity within an individual’s HES records.
There are slight differences when deriving an individual’s ethnicity using these different methods. Office for National Statistics published work has shown that using the modal (most common) methodology to assign an individual’s ethnic category in both GPES and HES reports higher agreement with Census 2021 ethnic recording when compared with using the recency (most recent) methodology. Using an individual’s most frequently recorded ethnicity within their records may therefore provide an ethnic category which better matches that recorded in census, which is regarded as the highest quality ethnicity data available.
The study design that analysts implement may influence their decision on which methodology to implement to derive ethnic category. Using both modal and recency methodologies may be appropriate in different scenarios. For example, if a longitudinal design is being implemented which uses the entire record history of a patient, the modal methodology may be more appropriate. Whereas, implementing a cross-sectional design where you require details (e.g. ethnicity) on an individual at a specific time point (date closest to cross-sectional date) may lend itself to implementing the recency methodology. It is important to note that the modal methodology produced higher agreement with census recorded ethnicity, however. The analyst and team should therefore take this into consideration and make the most appropriate decision based on their research question.
Learning Point 3
Based on current evidence, we recommend using the modal methodology to assign an individual’s ethnic category when using GPES and HES data sources, because it reports higher agreement with the ethnic category recorded in census in individual-level analysis.
Consideration of the study design and ethical considerations of the modal methodology should be considered before deciding which methodology should be applied. But producing the highest quality and most reliable ethnicity data is of utmost importance to inform public health analyses.
The ONS provides code to derive the most common and most recent ethnic category within episodic data (GitHub: ONS-Health-modelling-hub). Further detail of the logic and decisions can be seen in the code. This code is meant to act as a guide and to be built upon and improved by other analysts. An example of an improvement could include applying the receny methodology in those with ‘unresolved’ (see examples below and ONS publication for definition of ‘unresolved’ case) cases after applying the modal methodology.
In the modal methodology, the most common ethnicity is selected without considering the date of the record or its data source. In the recency methodology, a data source hierarchy is applied to resolve conflicting recent ethnicities. For GP data, journal table ethnicity is taken over patient table ethnicity (differences described in Section 1). For HES data, ethnicity is taken from HES-APC, then HES-AE and then HES-OP. In the modal methodology however, no additional methodologies are applied to resolve conflicting modal ethnicities. This is something that could be built upon and improved in the future by other analysts. The detail on data source hierarchies is further expanded in future sections.
Descriptions and worked examples of the modal methodology logic
Descriptions and worked examples of the modal methodology logic include the following:
Modal methodology example 1
- Description: Most recent ethnic recording is Other Asian, but the individual has multiple previous ethnic recordings of the Indian category.
- Examples:
- Other Asian (01.03.2023)
- Indian (01.02.2023)
- Indian (17.08.2022)
- Indian (03.11.2021)
- Decision: Indian
Modal methodology example 2
- Description: Most recent ethnic recording is a residual category, but the individual has previous valid ethnic category recordings
- Examples:
- Unknown (15.05.2019)
- White Other (03.01.2019)
- White Irish (03.01.2019)
- White Other (11.11.2018)
- Decision: White Other
Modal methodology example 3
- Description: Most recent ethnic recording is White British, but the individual has an even number of multiple different ethnic recordings
- Examples:
- White British (20.04.2021)
- White Other (31.03.2021)
- White Other (26.06.2020)
- White British (24.05.2020)
- White and Asian (20.03.2020)
- White and Asian (15.07.2019)
- Decision: Unresolved (using the modal methodology, a most common ethnic category cannot be identified)
Modal methodology example 4
- Description: The only ethnic recording is Black African
- Examples: Black African (20.08.2018)
- Decision: Black African
Modal methodology example 5
- Description: Most recent ethnic recording is White and Asian, but the individual has multiple previous ethnic recordings of a residual category
- Examples:
- White and Asian (18.05.2021)
- Not Known (15.05.2021)
- Not Known (02.03.2020)
- Decision: Not Known
Learning point 4: Reallocation methodologies
Given the difficulties and ethical consideration required for handling missing, discordant, and/or residual categories (such as Not Known, Not Stated) of ethnicity data, there is currently no consensus on applying statistical methods to deal with these issues. One could argue that ethnic category data is likely missing not at random, and this therefore violates a commonly applied assumption regarding whether multiple imputation is a viable option to handle missing data. Further, arguments may be made as to whether imputing a social construct, such as ethnicity, is ethical. Even if so, which variables to use to predict or impute ethnicity in an imputation model, given the plausibility of variables which predict ethnicity, may be difficult.
As shown previously in this paper, the distribution of the number of people within the ethnic categories differs between GPES and HES. A higher proportion of people have Not Stated or Not Known categories within HES, compared with GPES. This has implications when ethnicity is an exposure of interest in epidemiological analyses because analysts will often remove these people from their analysis, effectively treating these categories as missing data. In the case of HES data, this could mean losing up to 20% of the overall sample. Furthermore, for certain ethnic categories, such as the Any Other Ethnic Group category, evidence has suggested there is likely over-coding of this ethnic group.
Therefore, non-parametric methods may be a reasonable starting point for dealing with some of these issues. Individual-level analysis published by the Office for National Statistics shows the effect of reallocating certain ethnic categories and has reported that reallocating Not Stated and Not Known categories increases the number of people (the coverage) with a stated ethnic category, while only marginally reducing the agreement with the ethnic category recorded in census for some ethnic groups. This increase in coverage was particularly pronounced in HES data (Table 2). The pattern of findings was similar when applying the reallocation methodology to both the most recent and most common methods to derive ethnic category (above section).
Table 2. Impact of reallocation methodologies on coverage | ||
Linked dataset | Count of people in linked dataset with a stated ethnicity in both health and Census sources | |
Millions (n) | Percentage of the population of England on Census Day 2021 (%) | |
Linked census-GPES recency, no reallocation | 42.2 | 74.7 |
Linked census-GPES modal, no reallocation | 40.1 | 71.0 |
Linked census-GPES recency, Unknown only | 42.2 | 74.7 |
Linked census-GPES modal, Unknown only | 40.1 | 71.0 |
Linked census-GPES recency, Unknown and Any Other Ethnic Group reallocated | 42.2 | 74.7 |
Linked census-GPES modal, Unknown and Any Other Ethnic Group reallocated | 40.3 | 71.3 |
Linked census-GPES recency, Unknown, Not Stated and Any Other Ethnic Group reallocated | 42.6 | 75.4 |
Linked census-GPES modal, Unknown, Not Stated and Any Other Ethnic Group reallocated | 41.1 | 72.8 |
Linked census-HES recency, no reallocation | 37.2 | 65.9 |
Linked census-HES modal, no reallocation | 36.7 | 65.0 |
Linked census-HES recency, Unknown only | 39.7 | 70.3 |
Linked census-HES modal, Unknown only | 40.1 | 71.0 |
Linked census-HES recency, Unknown and Any Other Ethnic Group reallocated | 39.5 | 69.9 |
Linked census-HES modal, Unknown and Any Other Ethnic Group reallocated | 40.0 | 70.8 |
Linked census-HES recency, Unknown, Not Stated and Any Other Ethnic Group reallocated | 43.7 | 77.4 |
Linked census-HES modal, Unknown, Not Stated and Any Other Ethnic Group reallocated | 43.4 | 76.8 |
Source: Quality of ethnicity data in health-related administrative data sources from the Office for National Statistics
This table reports the number of people with a stated ethnicity after applying sequential reallocation methodologies. It does not report the agreement. For data regarding agreement, please see Figures 3 and 4 in our previous ONS analysis.
Learning Point 4
This learning point is dependent upon the research question at hand. If the aim of the study is, for example, a national-level analysis where maximum coverage of diverse populations is important, reallocation methodologies will increase the number of people with a stated ethnic category and therefore the overall sample. Applying more levels of reallocation does marginally decrease agreement with census recorded ethnicity for most ethnic groups, however. Therefore, there is a consideration between coverage and accuracy. Within HES, applying reallocation methodologies for ethnic categories significantly increases the number of people with a stated ethnic category and may therefore be more relevant to analysts using HES data. Within GPES data, applying reallocation methodologies does increase the number of people with a stated ethnic category, but less so.
Based on the findings of the Quality of ethnicity data in health-related administrative data sources, England: November 2023 publication from ONS, reallocating the Not Known and Not Stated categories as a default position may be an appropriate option. Reallocation of the Not Stated ethnic group would be at the discretion of the analyst given the ethical consideration on whether a Not Stated ethnic category can be treated as a ‘refusal’. It is important to recognise that while there are ethical considerations, producing the highest quality and most reliable ethnicity data is of utmost importance to inform public health decisions and public understanding. Reallocating the Not Stated category increases the coverage in both HES and GPES significantly. Qualitative work investigating the practices in recording ethnic category report that the practices for how the Not Stated and Not Known categories are completed by staff is inconsistent, and may result in lower quality of ethnicity data collection. Further reallocation of categories, such as Any Other Ethnic Group, Other White, Other Black, Other Asian, or Other Mixed may also be appropriate, but would be dependent on the research question and be at the discretion of the analysts. Future work further investigating the over coding and reallocation of these categories would be useful.
Analysts may also implement a hierarchy for ethnic categories if they decide to reallocate ethnic categories. This would allow analysts to make an analytical decision when conflicts in ethnic recordings occur (e.g. recordings on the same date, categories with the same number of recordings). We provide examples for the recency and modal reallocation methodologies where the hierarchy below was applied. Code for reallocating ethnic categories within episodic data can be found on the GitHub: ONS-Health-modelling-hub.
Example ethnicity category hierarchy in code and pop outs
-
-
-
-
- All ethnic categories other than Any Other Ethnic Group, Not Known and Not Stated
- Any Other Ethnic Group
- Not Known, Not Stated
-
-
-
Worked examples of reallocating Not Known, Any Other Ethnic Group and Not Stated categories, for modal methodology
Worked examples of reallocating Not Known, Any Other Ethnic Group and Not Stated categories, for modal methodology:
Reallocating example 1 – Modal
- Description: The most common ethnic recording is Not Known. The individual has a single previous ethnic recording category of Chinese
- Examples:
- Not Known
- Not Known
- Chinese
- Decision: Chinese
Reallocating example 2 – Modal
- Description:The modal ethnic recording is Not Known. The individual only has previous ethnic recordings of residual categories
- Examples:
- Not Known
- Not Known
- Not Stated
- Decision: Not Known
Reallocating example 3 – Modal
- Description: Modal ethnic recording is Not Known. The individual has multiple previous ethnic category recordings of Chinese and Indian with equal counts
- Examples:
- Not Known
- Not Known
- Chinese
- Indian
- Indian
- Chinese
- Decision: Unresolved
Reallocating example 4 – Modal
- Description: Conflicting modal ethnic recordings, all of which are residual categories
- Examples:
- Not Known
- Not Known
- Not Stated
- Not Stated
- Decision: Unresolved
Reallocating example 5 – Modal
- Description: Modal ethnic recording is Any Other Ethnic Group. The individual has previous ethnic category recordings of Chinese and Indian.
- Examples:
- Any Other Ethnic Group
- Any Other Ethnic Group
- Any Other Ethnic Group
- Chinese
- Chinese
- Indian
- Decision: Chinese
Reallocating example 6 – Modal
- Description: Modal ethnic recording is Not Known. The individual has previous ethnic category recordings of Any Other Ethnic Group, Chinese and Indian
- Examples:
- Not Known
- Not Known
- Not Known
- Not Known
- Any Other Ethnic Group
- Any Other Ethnic Group
- Any Other Ethnic Group
- Chinese
- Chinese
- Indian
- Decision: Chinese
Reallocating example 7 – Modal
- Description: Conflicting modal ethnic recordings, where one instance is Not Known and the other is Any Other Ethnic Group.
- Examples:
- Not Known
- Not Known
- Any Other Ethnic Group
- Any Other Ethnic Group.
- Decision: Any Other Ethnic Group
Worked examples of reallocating Not Known, Any Other Ethnic Group and Not Stated categories, for recency methodology
Worked examples of reallocating Not Known, Any Other Ethnic Group and Not Stated categories, for recency methodology:
Reallocating example 1 – Recency
- Description: Most recent ethnic recording is Not Known. The individual has previous ethnic category recordings of Chinese and Indian
- Examples:
- Not Known (25.02.2021)
- Chinese (17.06.2020)
- Indian (01.11.2019)
- Decision: Chinese
Reallocating example 2 – Recency
- Description: Most recent ethnic recording is Not Known. The individual only has previous ethnic recordings of residual categories.
- Examples:
- Not Known (25.02.2021)
- Not Stated (01.11.2019)
- Decision: Not Known
Reallocating example 3 – Recency
- Description: Most recent ethnic recording is Not Known. The individual has previous ethnic category recordings of Chinese and Indian on the same day.
- Examples:
- Not Known (25.02.2021)
- Chinese (01.11.2019)
- Indian (01.11.2019)
- Decision: Unresolved
Reallocating example 4 – Recency
- Description: Conflicting most recent ethnic recordings, all of which are residual categories
- Examples:
- Not Known (01.12.2019)
- Not Stated (01.12.2019)
- Decision: Unresolved
Reallocating example 5 – Recency
- Description: Most recent ethnic recording is Any Other Ethnic Group. The individual has previous ethnic category recordings of Chinese and Indian
- Examples:
- Any Other Ethnic Group (25.02.2021)
- Chinese (17.06.2020)
- Indian (01.11.2019)
- Decision: Chinese
Reallocating example 6 – Recency
- Description: Most recent ethnic recording is Not Known. The individual has previous ethnic category recordings of Any Other Ethnic Group, Chinese and Indian
- Examples:
- Not Known (25.02.2021)
- Any Other Ethnic Group (30.12.2020)
- Chinese (25.04.2020)
- Indian (17.06.2020)
- Decision: Chinese
Reallocating example 7 – Recency
- Description: Conflicting most recent ethnic recording, where one instance is Not Known and the other is Any Other Ethnic Group
- Examples:
- Not Known (25.02.2021)
- Any Other Ethnic Group (25.02.2021)
- Decision: Any Other Ethnic Group
Where a person only had Not Known and/or Not Stated and Any Other Ethnic Group categories recorded, Any Other Ethnic Group was prioritised and chosen as the reallocation destination.
Learning point 5: Hierarchy of data sources
Evidence has shown that there are differences between GPES and HES ethnicity recording in their agreement with census ethnicity recording. Furthermore, evidence has shown that there are differences within source, for example, differences in agreement when investigating HES subsets individually (specifically. HES-Admitted Patient Care [APC]; HES-Accident & Emergency (AE)/Emergency Care Data Set [ECDS]; HES-Outpatients [OP]). Therefore, it is important to understand how these differences may affect potential analyses, and if an analyst has both GPES and HES data sources available to them, it will impact which sources they should prioritise for deriving ethnicity.
Not all analysts have access to the same data. Analysts’ decisions will largely depend on the data sources they have available to them. For analysts that have full access to both GPES and HES data sources however, it is important to understand the ethnicity data quality and subsequent data source hierarchy.
NHS England has previously undertaken work and applied the below hierarchy of data sources to determine ethnic category. For analysts with full access to HES and GPES data sources, the previous hierarchy of data to derive ethnic category from was as follows:
-
-
-
-
- GP-Journal
- GP-Patient
- HES-APC
- HES-AE
- HES-OP
-
-
-
ONS has built upon the initial work by NHSE however, and the Quality of ethnicity data in health-related administrative data sources, England: November 2023 findings have updated the hierarchy. The updated hierarchy, based on the findings from the ONS analysis, is as follows:
-
-
-
-
- GP-Journal
- GP-Patient
- HES-APC
- HES-OP
- HES-AE
-
-
-
This hierarchy may also be used when there are conflicts of ethnic category on the same date or across data sources.
Learning Point 5
For analysts with both GPES and HES data available to them who are applying the recency methodology (or a new/hybrid methodology), applying the following hierarchy of data sources when deriving ethnic category data would be most appropriate:
- GP-Journal
- GP-Patient
- HES-APC
- HES-OP
- HES-AE
For individuals with only HES data available to them, apply the following hierarchy of data sources for ethnic category data:
- HES-APC
- HES-OP
- HES-AE
The hierarchy of datasets is dependent upon whether implementing the modal or recency methodology. The modal definition does not include a date or data source hierarchy (because it is taking the most common across all sources), therefore the dataset hierarchy does not apply to the modal methodology. Analysts may decide to implement a hierarchy within the modal methodology when taking this work forward to assess whether it influences ethnicity coding accuracy and completeness, however.
Worked examples of the dataset hierarchy, using the recency methodology
Dataset hierarchy example 1 – Recency
- Description: Individual has two different ethnic recordings on the same most recent date from two separate data sources
- Examples:
- Black Other (HES-APC, 19.05.2024)
- Black African (GP-Patient, 19.05.2024)
- Decision: Black African
Dataset hierarchy example 2 – Recency
- Description: Individual has two different ethnic recordings across three different data sources, two of which are on the same most recent date
- Examples:
- White Irish (GP-Journal, 28.05.2022)
- Other White (HES-APC, 28.05.2022)
- Other White (GP-Patient, 17.04.2022)
- Decision: White Irish
Dataset hierarchy example 3 – Recency
- Description: Individual has two different ethnic recordings across three different data sources
- Examples:
- White and Asian (HES-APC, 22.03.2018)
- Other Asian (HES-OP, 24.06.2017)
- Other Asian (HES-AE, 03.03.2017)
- Other Asian (HES-AE, 03.03.2016)
- Other Asian (HES-AE, 03.03.2015)
- Decision: White and Asian
Dataset hierarchy example 4 – Recency
- Description: Individual has three different ethnic recordings across three different data sources
- Examples:
- White British (GP-Journal, 17.06.2023)
- Other White (GP-Patient, 17.06.2023)
- White Irish (HES-APC, 17.06.2023)
- Decision: White British
Dataset hierarchy example 5 – Recency
- Description: Individual has two different ethnic recordings across three different data sources, two of which are on the same most recent date
- Examples:
- Indian (GP-Journal, 24.01.2022)
- Chinese (GP-Patient, 24.01.2022)
- Chinese (HES-APC, 22.01.2022)
- Chinese (HES-APC, 22.01.2022)
- Chinese (HES-APC, 22.01.2022)
- Decision: Indian
Dataset hierarchy example 6 – Recency
- Description: Individual has two recorded ethnic categories across two different data sources and dates
- Examples:
- Black Caribbean (HES-APC, 14.05.2023)
- Black Other (GP-Journal, 12.07.2022)
- Decision: Black Caribbean (while the Black Other ethnicity is from a higher hierarchy data source, it is not the most recent recording, which underpins the recency methodology)
Learning point 6: Missing ethnicity data
Currently, if a person has missing ethnicity data from their entire record history for GPES and/or HES sources, there is no consensus on how to assign an ethnic category for this individual. Based on current best practice, ONS analysis did not impute an ethnicity for individuals with missing ethnicity data within GPES and/or HES. This may change in the future as the evidence base develops and methodologies are advanced, however. If analysts have access to multiple sources of ethnicity data, maximising the sources available to them and linking to use ethnicity from the different data sources, may be appropriate, rather than having missing ethnicity data (which links to the above sections). Linking data needs to be done in a consistent, reliable, and ethical way, however. The UK Statistics Authority (UKSA) has held public consultations on public acceptability on handling ethnicity data, with the responses noted in the National Statistician’s Data Ethics Advisory Committee (NSDEC) Minutes and Agenda – April 2023 – UK Statistics Authority. Further work within ONS and partner agencies is also being conducted in this research area, within the context of our broader strategic aim. This aim is to explore the use of administrative data to produce population statistics, including characteristics, such as ethnicity. This work includes our Developing admin-based ethnicity statistics for England and Wales: 2020 article, which utilises a range of different administrative data sources available for population statistics
The ONS has also previously commissioned a technical review investigating methods for missing data. The technical review may provide a solid grounding for deciding on methodologies to deal with missing ethnicity data. Further resources into potential methodologies for imputing ethnicity data has been published by the SOA Research Institute and ONS Methodology team.
Learning Point 6
There is no consensus on how to handle missing ethnicity data within GPES and HES. More research is required on developing appropriate methods to manage missing ethnicity data within health administrative data sources.
Summary and concluding points
This paper has discussed issues which can affect analysts who are directly working to derive ethnic category in a dataset or working with ethnicity data in an analysis.
The main points discussed in this paper are strategies for:
- understanding the differences in ethnicity data in GPES and HES
- the aggregation and disaggregation of ethnic categories
- how to allocate an individual an ethnic category in episodic electronic health record data (meaning multiple recordings within their record history)
- the appropriateness of reallocating ethnic recordings and how to reallocate ethnic categories
- the hierarchies of GPES and HES sub-data sources
- missing ethnicity data
The learning points and examples provided are based on a funded project from the Wellcome Trust in collaboration with the Office for National Statistics (ONS). They were implemented as a starting point for a preliminary piece of research to provide a better understanding of the quality of ethnicity recording in health administrative data sources. It is acknowledged that the methodologies implemented in this project may not be applicable to all research projects, analytical situations or data sources, and all learning points, and examples provided are aimed to be built upon and improved by others who are working in this research space. This paper has been designed to be a working document, and the research community should work together to improve the learnings provided.
This paper only discusses analytical scenarios and situations for analysts working directly with ethnicity data. It is acknowledged that improving the quality of ethnicity data in administrative health data sources is a multifaceted problem. It requires a coordinated approach from many different organisations to improve the quality of ethnicity data. For example, collecting ethnicity at one point in time which spans across multiple different data sources and updating it periodically, rather than having many different datasets which collect ethnicity at multiple points in time, may be a more robust method to collect and process ethnicity data. Further, this paper does not touch upon the data collection methods for ethnicity data and there are likely to be improvements in the way that ethnicity data is collected in different healthcare environments. In addition, ethnicity is a social construct and can change over time or generations, therefore accounting for these factors and having flexibility built into the methods for collecting and processing ethnicity data is required.
Finally, while it is important to aim for systemic improvements in ethnicity coding practices, it is also important for analysts to follow good practice when handling ethnicity data. Irrespective of how analysts derive ethnicity data or group together ethnic groups within their data, they must ensure that they:
- assess data quality and biases of their chosen data source thoroughly and cross-validate against similar data sources
- provide evidence of how any miscoding has been addressed in their analysis
- communicate caveats in any reports or manuscripts
- interpret results with caution
- consider caveats when drawing any conclusions or recommendations
Acknowledgements
We acknowledge and thank the Wellcome Trust for funding this paper and wider project.
We also thank the Expert Review Panel for this project who provided expert opinion in the data sources, research design, interpretation of findings and dissemination and implementation plans. We also thank ONS colleagues who reviewed this document.
There are many other people and organisations, past and present, who are working to improve the quality of ethnicity coding, some of which we list below. We urge researchers, policymakers and officials who are interested in this research area to speak to these organisations and engage with them if they aim to improve the standard of ethnicity coding within UK health administrative data sources.
Additional resources
- Standards for ethnicity data – GOV.UK (www.gov.uk)
- Ethnicity harmonised standard – Government Analysis Function (civilservice.gov.uk)
- Diversity in Data | UKHDRA (ukhealthdata.org)
- GDPPR_Analytical_Code/Ethnic_Category at main · NHSDigital/GDPPR_Analytical_Code · GitHub
- Administrative Data Research UK. Data-driven change – ADR UK
- General Practice Extraction Service (GPES) Data for pandemic planning and research: a guide for analysts and users of the data – NHS England Digital
- Method for assigning ethnic group in the COVID-19 Health Inequalities Monitoring for England (CHIME) tool
- Hospital Episode Statistics (HES) – NHS England Digital
- General Practice Extraction Service (GPES) Data for pandemic planning and research: a guide for analysts and users of the data – NHS England Digital
- NHSDigital SNOMED CT Browser (termbrowser.nhs.uk)
- Quality of ethnicity data in health-related administrative data sources by sociodemographic characteristics, England Articles – Office for National Statistics (ons.gov.uk)