New is always better?
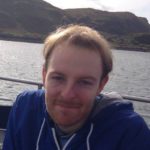
“New is always better!”, so says Barney Stinson in the long running comedy ‘How I Met Your Mother’, encouraging his friends to constantly move on and try new things. The joke being that his friend Ted wants nothing more than to find his soulmate and spend the rest of his life with the same person.
To shoehorn this analogy into statistics and methodology, our goal is to do everything in the best way possible, whether it’s data linkage, imputation, coverage estimation and adjustment, or disclosure control. Once you’ve found which method works best, you should stick with it and move on to solve another problem, right? Except, over time things change, and new developments arise.
We have a responsibility to try all the methods available to us and select the best for our outputs. We have a responsibility to try new things. Differential privacy has gained a lot of attention recently as a method for disclosure control (protecting confidentiality/privacy of respondents) and has been selected by the US Census Bureau as their means to protect the confidentiality of responses.
Statistical organisations must keep people’s data safe, and not disclose information about individuals. This is key to holding public trust. Differential privacy is hard to fully explain without an equation and some handy graphs, but the idea is essentially that if you were to add or remove any one person from a dataset, you wouldn’t notice a change in the outputs. It assumes a worst-case scenario in terms of the risk, and the most basic form can be summarised in one parameter, ε (epsilon) which is useful for comparing different levels of risk between approaches. Our usual approach is to consider whether individuals could potentially be identified in our outputs, and either reduce the detail given, or make changes to introduce uncertainty around those most likely at risk.
We ran a differential privacy pilot study, producing outputs on mortality data using two methods in the Secure Research Service.
We found that these methods provide good protection against disclosure, but although it can produce data which is very safe, it can also reduce the usefulness of the data more than traditional methods, in some cases introducing a bias. Some forms of differential privacy are also very computationally difficult to apply when there is a large number of variables. Based on the pilot, we don’t recommend the use of differential privacy, though there is a lot of research underway to overcome these issues and improve the method. In the future we may choose to use differential privacy over the ‘traditional methods’, and if we do, it won’t be just because it’s new.
The Office for National statistics (ONS) website has published the research paper “Applying differential privacy protection to ONS mortality data, pilot study” which provides more details on our findings.
If you’d like to talk about differential privacy or disclosure control more generally you can email sdc.queries@ons.gov.uk.