Estimating population by time of day
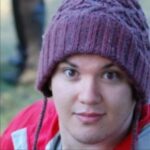
Imagine you were deciding where to build flood defences before a major storm hits in the afternoon. How would you choose where to build the defences to protect as many people as possible? Or imagine you were deciding where to open a new restaurant. How would you choose a location that gets the largest number of potential customers walking past your doors in the evening? In both cases you need to know where the population is at a particular time of day to make an effective decision. Our aim at the Office for National Statistics (ONS) is to enable these sorts of decisions through our transformed statistical system, and the first step to doing so is our innovative and experimental daytime population statistics.
ONS population statistics typically estimate the number of people who live in an area. But that’s only part of the information that users of our statistics need to know. Beyond estimating usual residents, it is really important to understand the number of people present in a local area by time of day to enable effective planning across the public and private sectors. Importantly, to accurately estimate the “population present” in an area we need to include both residents who are not temporarily absent and visitors who are temporarily present.
The ONS Methodology and Quality Directorate has been developing methods for estimating small area population size by time of day as part of our research on the future of population and migration statistics. We have just published our “Estimating population by time of day” case study that documents the progress we have made so far.
We used a range of data in a modelling framework called Population 24/7 to produce daytime population estimates for 14 Local Authorities in England and Wales. These experimental daytime statistics estimate the size of the local population at 2pm on a typical weekday during academic term time in 2019. These statistics give us new insight into where the population is during a typical working day, and in future could be used to inform a wide range of decision-making in government and beyond.
Compared to our mid-year population statistics that estimate the size of the usually resident population, our experimental daytime statistics show the population moving away from residential areas and into non-residential areas as people partake in daytime activities like work and school. This is particularly evident in urban areas. We can then map out the busiest areas for each Local Authority during the daytime. In future we will also show when those areas are busiest.
We have also developed a second set of experimental daytime statistics by working with the ONS Data Science Campus and using O2 Motion, which is Virgin Media O2 Business’ anonymised aggregate crowd movement data platform. These data estimate aggregate footfall in an area from the number of interactions between mobile phone handsets and masts. We have used these mobile-derived statistics as a first attempt to validate our model-based statistics. This is the first time that mobile phone data have been used by ONS to produce daytime population statistics, and this is a radical step forward in the way we use innovative sources of data to serve the public good.
Our results are promising, and there is much more work to do. In the future we will be developing methods to quantify the quality and uncertainty of our daytime statistics. We will also be improving the coverage of different kinds of population mobility. We will be investigating:
- retail activity
- leisure activity
- non-motorised transport
- rail travel
We will also improve the use of crowd movement data and other novel sources of high-frequency low-latency data to make our daytime statistics as timely and accurate as possible.
If you are interested in learning more about this research, please contact us at MQD.Engagement@ons.gov.uk.