Chicago machine learning . . . and all that jazz!
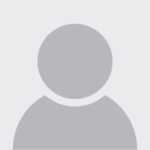
As a new starter to the Office for National Statistics, (ONS) I’m on a mission to grow. One part of that mission is to become a better data scientist by tackling Machine Learning (ML), a branch of data science which focuses on the use of data and algorithms to imitate the way that humans learn. Not for the faint-hearted or so I thought, but there’s no shortage of support in the Methodology and Quality Directorate. Shortly after I started in July, I was invited to take part in an ML and economics discussion group. The following is a short summary of what the group did, the start of my learning journey, and what comes next.
The sessions were a review and discussion of pre-recorded lectures on ML in economics. Maybe not the Netflix binge you’d imagine, but this turned out the be a real booster of a learning experience. We reviewed ten sessions over the course of ten weeks from the Chicago Booth Centre for Applied Artificial Intelligence. and our group was a mix of methodologists, economists, and data scientists. As a newbie with a social science background, I was not a natural fit for the group, but I felt welcomed, and this wide-ranging group of experts gave back a rich mix of technical and philosophical discussion.
So, what did we cover, how did we digest it, and what interested me throughout? As mentioned, these were a series of summer school lectures focused on ML in economics and were a mix of technical detail, context, and applied examples. Our roundtables covered what we liked, didn’t like, could apply in our own research, and any other interesting observations.
What came out of the videos could be split into two parts: for me, the newbie, a useful set of resources and introductions to ML; for our more expert members, a blend of interesting, insightful, technically challenging and critique worthy looks at ML for economics in action. ML feels like it’s evolving, but with us and not against. We saw some fascinating examples of how it has progressed beyond “just complicated stats”, providing real application and insight. For example, did you know ML models:
- Can be used with satellite imagery showing luminosity (how much a city is lit up at night) to identify the GDP of a country?
- Have used the distribution of cattle in rural areas of Africa to fast track Covid-19 financial support?
- Can learn unwanted biases? We saw this in action from a recruitment example selecting candidates from their CV, and, more importantly, also how to overcome these biases.
- Are used to aid and better inform life impacting decisions such as who gets a heart operation or who gets bail from prison?
- Can be used to learn about behavioural intentions through text analysis? For example, by analysing tweets.
- Can help qualitative analysts by learning their needs and processing larger data sets on mass and at speeds we can’t. So, ML with, not replacing, human insight.
But let’s not be naïve here. ML is not without its room for growth or debate. We challenged whether there was enough economics in these sessions. We repeatedly sense-checked whether this work builds or loses public trust in stats. And talked bias. A lot. Bias in the data we receive, and the knock-on for the data we produce. And we also continuously asked ourselves where this new learning can be applied.
My philosophy is ‘interesting is good, but useful is better.’ Not only has this group had that philosophy, but it’s also given me a great deal of ‘useful’ to work with. A useful resource to learn from, an engaging shared knowledge base and experience, and a network of experts and learners to grow with.
So, what’s next on the agenda for me and the group? We are working on the next round of view and review sessions for anyone interested in ML across the GSS. These will be suitable for people at any stage of their learning. If you’re interested in taking part and have any suggestion, questions or input please contact the Data Science and High-performance Computing (DaSH) expert group via DaSH@ons.gov.uk or feel free to get in touch with me and share your thoughts via graham.brennan@ons.gov.uk.
If you would like to know more about the research that is ongoing in the Methodological Research Hub or across the Methodology division, or if you are interested in collaborating, please contact Methods.Research@ons.gov.uk.