Building theoretical as well as technical capability
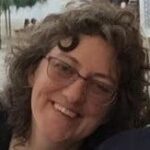
Recently, the Natural Language Processing Working Group (NLPWG) at the Office for National Statistics (ONS) invited Dr Aaron Reeves, Associate Professor of Evidence-Based Social Intervention and Policy Evaluation at the University of Oxford’s Department of Social Policy and Intervention, to answer some questions we had about topic modelling. Topic modelling is a way of grouping free text into distinct themes. Dr Reeve’s approach and the group discussion it encouraged made me realise that we often get too caught up on the technical question of how to do something, and ignore the more theoretical question of why we are doing it. Statistics exist in a context and understanding that context helps us to not only interpret the results, but to develop our tests more accurately.
The NLPWG is based in the Methodology and Quality Directorate (MQD), in the ONS. The group was established in 2021 to explore use cases and to develop methodological best practice in Natural Language Processing (NLP) for official statistics. ONS deals in a vast amount of free-text data that comes from surveys, focus group transcripts and publications. ONS has a great deal of qualitative expertise. But qualitative methods can often take a lot of time and resource to implement. There are some processes that can be automated so that qualitative analysts can spend more time on the tasks that need more specific methods or interpretation.
The NLPWG has just over 20 members from different ONS areas and has contributed to projects and publications on:
- attitudes to COVID-19 lockdown
- attitudes to climate change
- reasons for COVID-19 vaccine hesitancy
- research into biases in occupational coding and data linkage
- identifying duplication and overlap for a survey transformation
- automating horizon scanning to build capability for emerging methods
- exploring the effects of negative language
Our projects are a combination of business requirements and ‘blue skies’ research that meets the Office’s strategic objectives. Members are volunteers who agree to give some of their time to build NLP and coding skills. We have a GitLab repository and work is peer-reviewed for both methodological robustness and coding best practice. We offer mentoring and shadowing to less experienced members and we can allocate ‘simpler’ coding tasks to help build people’s knowledge and confidence. This could include things like the opportunity to work on reproducible data ingestion code.
The skillset of the audience at the session with Dr Reeves was very varied, so I asked him to give a quick overview of structural topic modelling. I had wrongly assumed that he would concentrate on specific statistical packages or code. But he chose to introduce the topic on a theoretical perspective instead. He spoke about how people use language and how it can be categorised to improve meaning. Afterwards he explained how topic modelling can be used to facilitate this on a large scale.
By introducing the work in less technical terms Dr Reeves was able to engage the non-technical users as well as encouraging the more experienced members of the audience to think about the topic in a new way. This approach made me see that it is easy to forget the meaning behind our work when we are under pressure to produce work quickly or when we are excited to learn something new. But while it is important to know how a method works or when a method has been unsuccessful, it is also important to understand why you might be seeing a certain type of results. This is an important part of being able to interpret results successfully.
It is also important to understand why people might behave in a particular way. This can help you plan your analysis before you start. Language is cultural. If you ignore the nuances of why people might communicate in a particular way it can negatively affect the quality of your work.
So, what happens next? We left the meeting offering Dr Reeves membership to the NLPWG. He is going to put us in contact with other academics with whom we might develop beneficial relationships. I’m planning a quarterly external ’question and answer’ session and have already invited another academic to talk to us about normative language and the effect of unconscious bias. I’m also planning a panel session in for 2023 with at least two speakers. While we already encourage analysts to share interesting articles, each project in the Git repository will have a section for relevant literature. This means that anyone using the code can also see the underlying constructs.
I know it’s easy to get caught up in the excitement of a new method or new project. But try not to forget why we’re using that method. Having the background knowledge helps us to engage staff, customers, and other stakeholders and shows us other opportunities to use the methods. With an increasing number of new ‘cool’ statistical and data science techniques and data, it’s more important than ever that we understand the methods we use, not just the ways to run them.